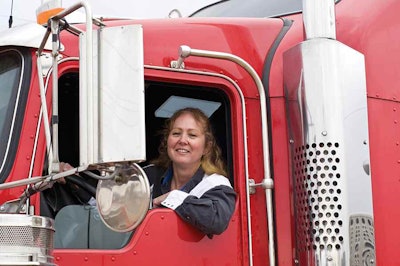
Early indicators of driver turnover are in data, but they are not always on the surface like weekly fluctuations in pay or work schedules. The more useful information is often buried in patterns of data that show a change in behavior and attitude.
Trimble Transportation’s Vice President of Data Science Chris Orban has many years of experience developing predictive driver turnover models. He recently visited with CCJ to provide an update on a new capability for Trimble’s Driver Retention model.
The algorithms used by the Driver Retention model have, to-date, relied on purely objective data, he said. The data comes from telematics systems and transportation management software (TMS). Hours-of-service and operational data is used by the model to predict if drivers are getting good sleep, working efficiently and getting home on time.
Orban said driver messaging is already used as a predictor for turnover. If a driver sends more messages than normal he or she may be experiencing a concern or disruption, he noted. Likewise, a driver who sends too few messages is likely “disconnecting and not really focused on the job or company.”
The predictive model is now adding “sentiment analysis” to identify drivers who have an elevated turnover risk based on changes in their moods and feelings. Sentiment analysis combines the words drivers send in messages in the predictive model with operational data to understand the context in which the words are used, he explained.
As an example, a driver might send a fleet manager a message to complain about a certain shipper. The model could determine the context of the message. Operational data may show the driver had been dispatched to the same customer three times in a row. The message would therefore carry more weight for dissatisfaction and turnover intent.
Likewise, a driver could be dispatched on three consecutive loads with below-average mileage. With sentiment analysis, the model might detect a change in a driver’s message tone, even if a driver didn’t complain specifically about mileage.
Overall, the new capability will give fleet managers a better understanding of changes in driver behaviors, Orban said, and will present information directly within a TMS to help address those concerns proactively to change the outcome.
A retention score is displayed within a TMS to give a fleet manager or load planner information to make decisions. A load planner may decide to not put a certain driver with a low retention score on a load that would further exacerbate a problem, for example.
The Driver Retention model also connects with a new driver-load matching optimization tool from Trimble Transportation called Dispatch Advisor.
“We are looking at each load for each driver and how that would impact retention,” Orban said.
Trimble revises the algorithms of the Driver Retention model on a monthly basis. An example of how the model continues to adapt and learn is the capability to account for differences in how drivers communicate based on where they are from, or what type of operation they do, Orban noted.
Ultimately, the goal for any revisions are to identify the right thing to do with drivers at the right time, he said. The “right time” to have a conversation with drivers is when their sentiment begins to shift. When this happens, fleets have a narrow window of opportunity to communicate and influence their decision to stay.
Using a software system that analyzes driver messages to determine mood could seem invasive, Orban admitted, but stressed the reason fleets are willing to use technology to this extent is because they truly care about drivers and want to understand how they feel and what is going on in their work life.