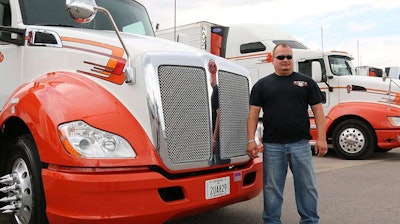
Ten years ago, a startup company named FleetRisk Advisors created a predictive model for Dupre Logistics. This was the first of its kind in the transportation industry.
The model analyzed hundreds, even thousands, of data elements to identify drivers who are most likely to be in accidents during the next few days and weeks. FleetRisk then created models to predict which drivers would file workers comp claims or quit.
As more fleets signed up the results got better. As more data fueled the analytical engines, more patterns in the data emerged as harbingers of future events.
The models also identified the countermeasures to stop risky events from happening. They come in the form of suggested topics for conversations between managers and drivers about personal, professional or financial issues that the data suggested drivers were having.
Most of the patterns identified by the models are not apparent just by looking at the raw data.
To date, experience has shown that use of predictive intelligence is more effective at preventing driver turnover than preventing accidents, says Dean Croke, one of the founders of FleetRisk Advisors. The reasons drivers quit are by and large the same, he says. Accidents by comparison are much more complex and far less repeatable.
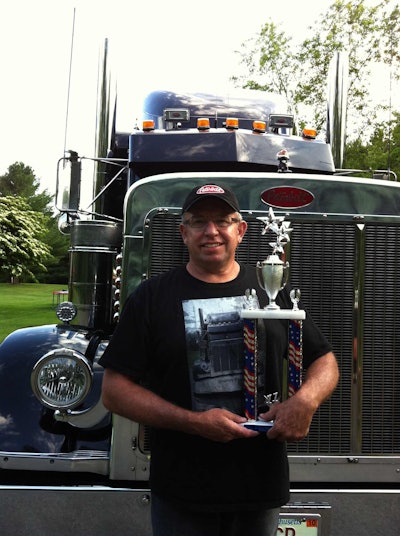
“Drivers are very predictable. They experience the same frustrations,” says Croke, who today is vice president of Omnitracs Analytics, the name given to the company after the purchase of Omnitracs and its subsidiary, FleetRisk Advisors, by Vista Equity Partners in 2013.
During a recent interview with CCJ, Croke shared some common predictors that surface for driver turnover. Each client has unique predictors but the early signs are often what you would expect drivers are frustrated with.
Fluctuations in pay and mileage, extra time waiting at docks to be loaded or unloaded, and moments when drivers request home time but do not get their requests fulfilled are common predictors that come from running fleets’ operational data through its algorithms.
One predictor you might not expect is the state that drivers have their CDL license from. The likely explanation is that over-the-road drivers in states that have comparatively low freight volumes find it more difficult to get home as regularly as others. Naturally, they experience more frustration.
“It’s obvious from the start that the driver is going to quit,” he says.
On the flip side, the patterns in data can also explain why drivers stay. Drivers that stay longer have a markedly different profile than those who quit given the same set of circumstances. By identifying common traits in driver profiles, Croke says it is possible to know the probability a new job applicant will stay or leave.
Using predictive intelligence to make hiring decisions is a challenge, however, since fleets generally want to streamline their hiring process and not create additional hurdles for drivers who meet their minimum qualifications.
Even so, it is possible to identify drivers that are high risk of early turnover, and he suggests that fleets could spend extra time with those at their orientation training meetings to set the right expectations.
Omnitracs Analytics has un-bundled its products to address specific needs fleets have, he explained. Some may only want to score driver job applicants to identify those that are likely to quit early on. New products are also on the way, such as a model that would be used by drivers to identify carriers and the type of operation – flatbed, tanker, long haul, dedicated, etc. – that will most likely keep them satisfied for the long run.
Another future possibility is to incorporate more data about driver physiology, like sleep patterns, into the models to predict accidents and turnover. Croke, who is an expert in sleep science, believes that sleep deprivation is a reason why the driver turnover rate is the highest during the first 90 days on the job. It takes about 90 days for drivers to adjust to the new work and sleep schedules when they change companies, he says.
Being able to capture not just the quantity but the quality of drivers’ sleep is possible. Wearable devices can communicate with Omnitracs’ in-cab mobile devices through Bluetooth and provide a reliable stream of such data, he says. At some point, he imagines that predictive models will be recommending that fleet managers see to it that drivers have more opportunities to get a good night of sleep. It just might keep them around for longer.