Editor’s note: This is the third and final piece in the Predictive Analytics series. Part one covered the future of fleet maintenance and Part two looked at the progression of driver safety data.
According to data reported by the American Trucking Associations, driver turnover rates dropped to historically low levels — at least during the first part of 2017.
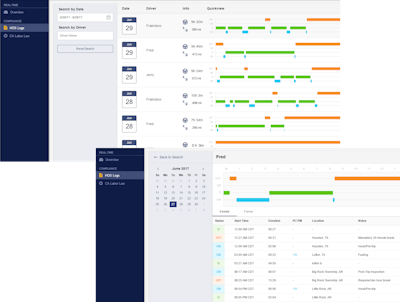
Truckload carriers with revenues of over $30 million had a 74 percent annualized turnover rate and smaller carriers had 66 percent. The trends may point to brighter days ahead for turnover, but a more useful statistic would be which drivers will leave, and why, to prevent it.
Just as predictive analytics are helping fleets avoid equipment breakdowns and accidents, as covered in parts 1 and 2 of this series, they can identify drivers who are the most likely to quit to stage an early intervention.
Driver surveys are perhaps the easiest way to identify the early signs of turnover, especially during critical periods of the driver employment lifecycle.
Research from Stay Metrics shows that 33 percent of new driver hires will leave their carriers within 90 days and an additional 22 percent will quit within 180 days. Stay Metrics administers driver surveys for motor carriers at the 7 and 45-day periods of employment to gather feedback on early job expectations, experiences, and satisfaction levels.
The surveys are sent to drivers as text messages with a link to an online form. Stay Metrics immediately notifies the carrier via email if a driver’s responses indicate a high degree of turnover risk. For instance, a driver may have unrealistic expectations for pay on the first week of the job.
The company also alerts fleets if drivers do not respond to the surveys in a timely manner. Stay Metrics research shows that drivers who do not respond to surveys are 48 percent more likely to leave, says Tim Hindes, chief executive officer.
Stay Metrics also administers an in-depth annual Driver Satisfaction survey for clients. The responses can be run through its predictive model to show what specific factors cause turnover at each company relative to peer groups for dry van, refrigerated, flatbed and other industry sectors.
Survey data is one of many possible data sources for predictive driver turnover models.
Maven Machines recently put the finishing touches on a new fleet management platform called SmartSense. The platform includes a wireless headset that captures head movements of drivers along with ELD and telematics applications.
SmartSense sends all of its mobile data capture to a cloud platform that brings in data from other sources, including weather and traffic, and fleets’ back-office IT systems through integration. The cloud platform processes “hundreds of millions of events every hour,” says Avishai Geller, chief executive officer.
All of this data processing creates a real-time predictive score for driver safety and performance, that accounts for the conditions the driver operated in. For example, if the driver was in bad weather, less head movements are better, Geller says.
The company is working on new predictive models for driver turnover. Today the mobile app for SmartSense has a feature drivers use to input home time requests that gives dispatchers visibility. The company is building algorithms to help dispatchers optimally schedule loads to meet home time commitments.
The company is also leveraging its data points to predict which drivers are at risk of leaving. The SmartSense platform can capture detention time, requested home time, driver pay information, and quality of equipment using inspection data.
“All of these are factors that go into driver satisfaction,” he says. “We are creating a platform that helps companies operate better, end-to-end, and driver retention is a huge pain point.”